MIT Research: Multi-Agent Debates Enhance AI Robot Intelligence
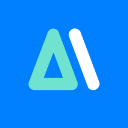
In today's digital world, the use of short text has become central to online communication. However, these texts often lack common vocabulary or context, posing numerous challenges for Artificial Intelligence (AI) during analysis. In response, Justin Miller, an English Literature graduate student and data scientist from the University of Sydney, proposed a novel approach that utilizes Large Language Models (LLMs) to gain deeper understanding and analysis of short texts. Miller's research focuses on how to analyze a vast array of short texts, such as social media profiles,
DeepSeek has officially released and open-sourced its latest large language model R1, which has shown outstanding performance and is considered comparable to OpenAI's official version o1. This initiative not only marks another significant breakthrough in domestic AI technology but also provides new options for AI developers worldwide. DeepSeek R1 has extensively applied reinforcement learning techniques in the post-training phase, significantly enhancing the model's reasoning capabilities even with minimal labeled data, in areas such as mathematics, coding, and natural language reasoning.
Recently, Zhejiang University and Alibaba DAMO Academy jointly released a remarkable study aimed at creating high-quality multimodal textbooks from educational videos. This innovative research not only provides new ideas for training large-scale language models (VLMs) but may also change the way educational resources are utilized. With the rapid development of artificial intelligence technology, the pre-training corpus of VLMs mainly relies on visual-text pairs and visually intertwined data. However, much of this current data comes from the web, where the correlation between text and images is weak, and the knowledge density is relatively low.
Recently, a study led by the Austrian Institute of Complexity Science (CSH) revealed that despite large language models (LLMs) excelling in various tasks, they show significant shortcomings when tackling advanced history questions. The research team tested three leading models, including OpenAI's GPT-4, Meta's Llama, and Google's Gemini, with disappointing results. Note: The image was generated by AI, and the image is licensed by Midjourney to evaluate these models in history.