Self-Supervised AI Model GedankenNet: Farewell to Real Data Feeding, Redefining Holographic Microscopy Reconstruction
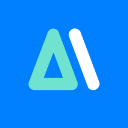
HyperAI超神经
55
The recently highlighted self-supervised AI model, GedankenNet, has garnered attention. Unlike traditional models, GedankenNet does not require real data for training. Instead, it learns through thought experiments and physical laws, bringing new hope to the field of holographic microscopy reconstruction. The model is trained using physical consistency loss, eliminating the need for iterative processes, resulting in faster speeds and higher accuracy. Research outcomes indicate its exceptional performance in image quality and external generalization, promising to advance the field of holographic microscopy and reduce reliance on large-scale data.
© Copyright AIbase Base 2024, Click to View Source - https://www.aibase.com/news/1567