Google Research: Large Language Models Struggle to Self-Correct Reasoning Errors
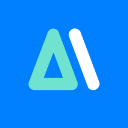
站长之家
36
The latest research from Google DeepMind indicates that large language models struggle to self-correct reasoning errors without external guidance. Researchers have found that when models attempt to correct their initial responses based solely on their own thoughts, they often make mistakes. The study also reveals that achieving self-consistency through multiple model votes can be achieved, but this falls short of true self-correction. The researchers emphasize that these findings are of significant importance for the development of applications requiring higher safety standards. They call for continued efforts to improve existing language models, grounded in an understanding of the potential and limitations of self-correction.
© Copyright AIbase Base 2024, Click to View Source - https://www.aibase.com/news/2265