Shandong University and the University of Electronic Science and Technology have developed the interpretable deep learning algorithm RetroExplainer for retrosynthesis prediction in organic chemistry. This algorithm can identify synthesis routes for target products within four steps, while also providing interpretable decision-making processes. Experiments show that RetroExplainer outperforms other algorithms on multiple benchmark datasets, with 176 experimental routes designed for 101 cases mostly having references in the literature, demonstrating its feasibility. This algorithm balances model performance and interpretability, serving as a tool for organic chemistry synthesis research and reflecting the development direction of interpretable AI.
Shandong University Develops Explainable Deep Learning Algorithm RetroExplainer, Identifying Organic Compound Retrosynthetic Routes in 4 Steps
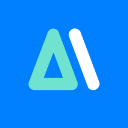
HyperAI超神经
This article is from AIbase Daily
Welcome to the [AI Daily] column! This is your daily guide to exploring the world of artificial intelligence. Every day, we present you with hot topics in the AI field, focusing on developers, helping you understand technical trends, and learning about innovative AI product applications.