Recent studies have revealed that GPT-4 performed poorly in a visual recognition challenge task, possibly because the images in this task were overly common in the training set, leading GPT-4 to rely on memorization rather than genuine visual recognition capabilities. This indicates that even large models that excel in certain tasks require careful evaluation; their success in the training set should not lead to an overestimation of their generalization abilities. Enhancing the model's generalization and robustness against adversarial samples remains a key research focus. It is also crucial to be wary of testing models solely on the training set; evaluating their generalization capabilities across a broader range of samples is essential for a more accurate assessment of model performance.
New Challenges for GPT-4 in Visual Recognition Tasks
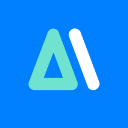
量子位
65
© Copyright AIbase Base 2024, Click to View Source - https://www.aibase.com/news/3154