LeCun Exposes Major Defects in Multimodal LLMs, Proposes Interleaved-MoF to Significantly Enhance Visual Understanding
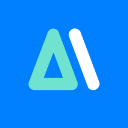
站长之家
This article is from AIbase Daily
Welcome to the [AI Daily] column! This is your daily guide to exploring the world of artificial intelligence. Every day, we present you with hot topics in the AI field, focusing on developers, helping you understand technical trends, and learning about innovative AI product applications.